5.8 Types of predictor variables
In the discussion so far, we have included multiple predictors in a model without making any explicit distinction between their roles. This section assumes there is a single primary predictor of interest for which you would like to test the association with the outcome. In this context, we discuss the distinctions between the roles of other predictors, which might be confounders, mediators, or moderators, and illustrate these distinctions using causal diagrams.
5.8.1 Confounder
In many studies, there is a primary predictor of interest and the goal is to obtain an unbiased estimate of the effect of that predictor on the outcome. The causal diagram, ignoring all other variables, is illustrated in Figure 5.7.

Figure 5.7: Association between predictor and outcome
However, especially in an observational study, there may be confounders – variables that are associated with both the predictor and the outcome and are not in the causal pathway from predictor to outcome, as illustrated in Figure 5.8.
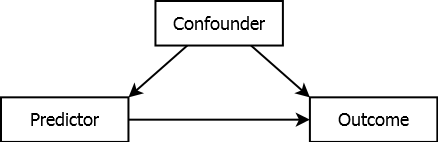
Figure 5.8: Confounded association
Suppose the confounder is positively associated with both the predictor and the outcome, but the predictor is not actually associated with the outcome. Failure to adjust for confounding may lead to the spurious (incorrect) conclusion that the predictor is associated with the outcome; differences in the outcome corresponding to differences in the predictor are actually due to differences in the confounder. When there is an association between the predictor and outcome, failure to adjust for confounding may result in not identifying that association, or over- or under-estimating it. Including the confounder in the regression model along with the predictor is an attempt to adjust for confounding. Confounding can be adjusted for in the study design (e.g., matching, randomization, restriction of the scope) or analysis (e.g., stratification, standardization, regression adjustment). In this text, we focus only on regression adjustment.
For example, suppose a researcher’s goal is to estimate the effect of weight loss on metabolic syndrome, defined as having at least three of the following five risk factors: (1) large waist circumference, (2) high blood pressure or taking blood pressure medication, (3) elevated triglycerides, (4) elevated fasting glucose or taking medication to lower glucose, and (5) low high-density lipoprotein (for specific cutoffs, see http://my.clevelandclinic.org/health/articles/metabolic-syndrome, accessed 1/4/2021). However, the effect of weight loss on metabolic syndrome may be confounded by income, as illustrated in Figure 5.9.
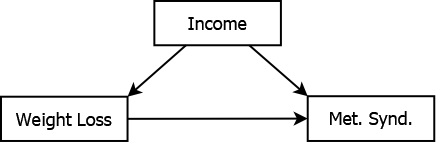
Figure 5.9: Income confounding the association between weight loss and metabolic syndrome
Individuals with fewer financial resources may find it more difficult to lose weight and also may be more likely to have poor metabolic characteristics. Thus, the effect of weight loss is confounded with the effect of income. In order to estimate the true (unconfounded) association between weight loss and metabolic syndrome in an observational study of individuals spanning a range of income levels, you need to adjust for potential confounding due to income. Failure to adjust for income may result in obtaining a biased estimate of association; part of the unadjusted “weight loss effect” may, in fact, be an “income effect.”
5.8.2 Mediator
A mediator is like a confounder in that it is associated with both the predictor and the outcome. However, unlike a confounder, it is in the causal pathway. When mediation is present, the predictor typically has both a direct effect on the outcome (not through the mediator) and an indirect effect (through the mediator), as illustrated in Figure 5.10. If you are interested in the total effect of the predictor on the outcome, regardless of the pathway, then do not adjust for a mediator; doing so would bias the predictor’s effect estimate by removing some of the effect you are actually interested in.
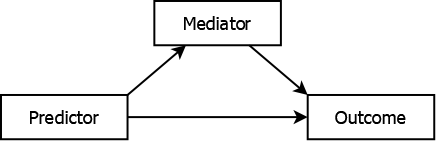
Figure 5.10: Mediated association
For example, adipocytokines may mediate the effect of weight loss on metabolic syndrome (Matsuzawa 2006; Rolland, Hession, and Broom 2011), as illustrated in Figure 5.11.
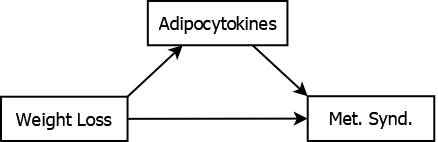
Figure 5.11: Adipocytokines mediating the association between weight loss and metabolic syndrome
Weight loss leads to an improvement in the characteristics that define metabolic syndrome, in part, due to its effect on levels of adipocytokines. Adjusting for adipocytokine levels would result in attenuating the estimate of the total effect of weight loss since you would be removing part of its effect (the indirect effect through adipocytokines). Therefore, if you are interested in the total effect of weight loss, do not adjust for adipocytokine levels. Compare this to the role of income as a confounder in Figure 5.9. Income precedes weight loss in the causal pathway – the effect of weight loss on metabolic syndrome is not through changes in income, but might be explained by differences in income between those who differ in weight loss. Thus, in these examples, income is a confounder while adipocytokine level is a mediator.
Another example is related to the study of health disparities. Should you “control for” socioeconomic status (SES) when studying racial disparities in health outcomes? In such a study, the health outcomes of individuals of different race/ethnicities are compared. “Race/ethnicity,” a social not biological construct, acts as a proxy for structural racism, the actual reason for disparities (American Medical Association 2020). In the U.S., SES is correlated with both race/ethnicity and health outcomes which may lead one to believe it is a confounder that should be adjusted for. However, SES is in the causal pathway between racism and health outcomes and therefore is a mediator of their association (see Figure 5.12).
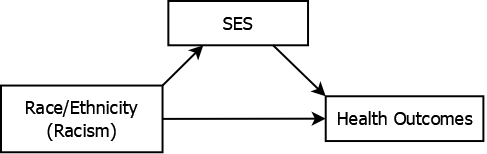
Figure 5.12: SES mediating racial disparities in health outcomes
If you “control for SES” you will remove part of the effect you are trying to estimate and underestimate disparities in health outcomes, perhaps even concluding there are no disparities. For example, Yehia et al. (2020), after adjusting for a number of demographic variables, conclude that race/ethnicity is not associated with COVID-19 mortality. However, Katikireddi et al. (2021) contend that this conclusion is in error exactly because the analysis adjusted for mediators. See also, for example, Meghani and Chittams (2015) regarding SES, and Zalla et al. (2021) and Schnake-Mahl and Bilal (2021) regarding the role of geography as a mediator of racial disparities in COVID-19 mortality.
Investigating the nature and magnitude of mediation, and decomposing the total effect into its direct and indirect components, is the realm of mediation analysis and is beyond the scope of this text (see, for example, Hayes (2022)). However, even if you are interested only in the total effect, it is vital to understand the distinction between mediators and confounders, and to not include mediators in a regression model — including a mediator will adjust out part of the very effect you are trying to estimate.
5.8.3 Moderator
In the above examples, there is a single predictor effect. In the case of confounding, the effect is obscured but there is still just one effect and the solution is to adjust for the confounder in the design or analysis. In the case of mediation, the effect is in part due to another variable but, again, there is still just a single effect of interest. Some variables, however, are moderators (or effect modifiers) – the effect of the predictor on the outcome depends on and varies with the level of the moderator. The predictor does not have a single effect, but rather a range of effects spanning the range of values of the moderator, as illustrated in Figure 5.13. The multiple lines going from Predictor to Outcome correspond to multiple magnitudes of association, with values that depend on the value of the moderator.
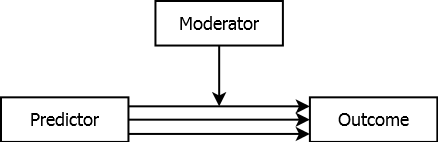
Figure 5.13: Moderated association
A moderator in a regression model is a term that is involved in an interaction (discussed in Section 5.9). In a regression model, include both the moderator and its interaction with the predictor.
For example, the effect of weight loss on metabolic syndrome may be moderated by baseline metabolic characteristics (see Figure 5.14) . Weight loss might have a greater impact among individuals with more room for improvement. By including the baseline measurement and a baseline \(\times\) weight loss interaction in the regression model, you can estimate how the weight loss effect varies between those with different baseline metabolic characteristics.
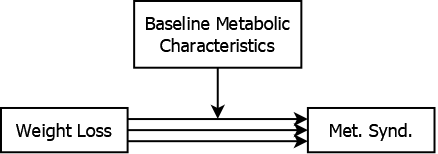
Figure 5.14: Baseline values moderating the association between weight loss and metabolic syndrome
Including a variable as a moderator also takes care of any confounding bias due to that variable, but in a different way than when including a confounder without an interaction. Including an interaction is similar to stratifying the analysis by another variable and estimating the effect of a variable within levels of another. If you stratify a regression analysis by a confounder that is not a moderator, then within each stratum you would get (approximately) the same effect. The regression coefficients would be approximately the same between strata (exactly the same in theory, but in practice they would vary, just not meaningfully). That single within-strata effect may be different than the effect if you ignored the confounder, but it would be the same within strata. By stratifying, you are removing the confounding – the confounder does not vary within strata so is no longer associated with the predictor or outcome within strata. When you “adjust” for a confounder in a regression, this is sort of what is happening, but mathematically its different than stratifying. If you were to stratify your analysis by a moderator, however, then you would get different effects between strata – the regression coefficient for the predictor would vary between strata.